AI – a Helping Hand in Primary Care?
Takeaways from a trial that applied AI to six years of patient data kept by a local healthcare centre in Sweden.
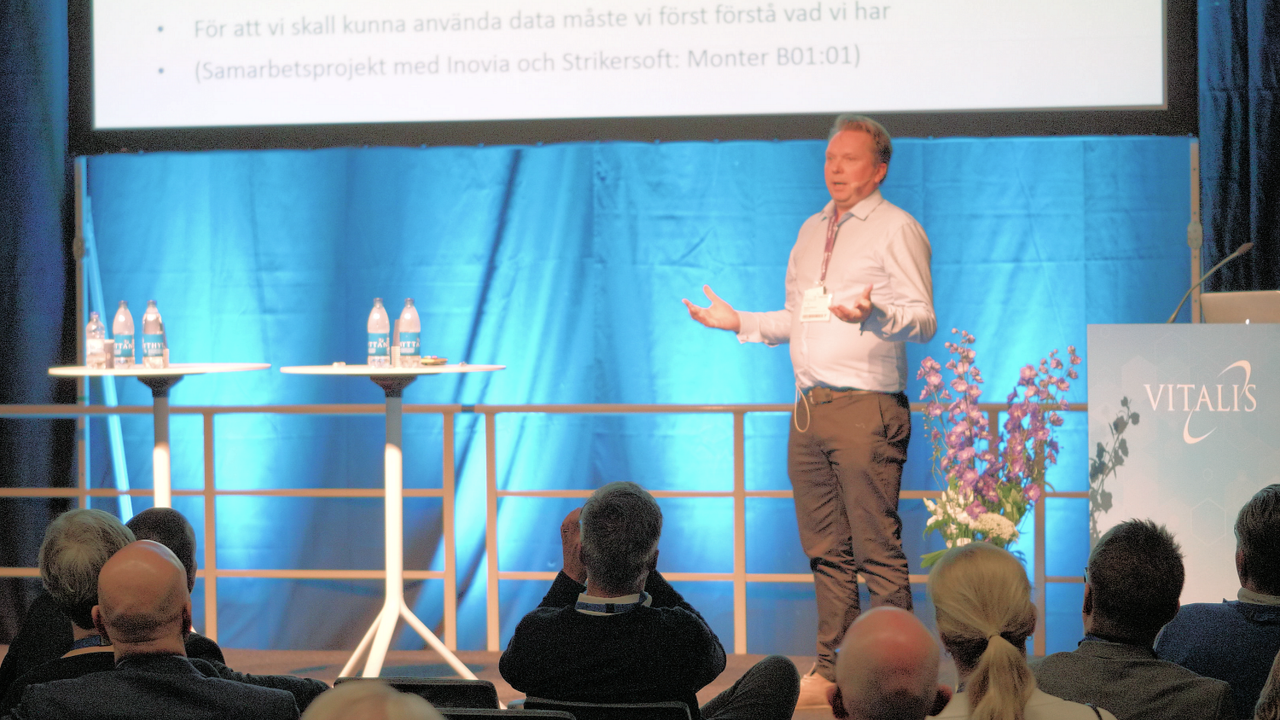
Fredrik Eriksson, Senior Medical Advisor at Strikersoft, recently gave a lecture on AI in healthcare at the Vitalis conference and exhibition held in Gothenburg, Sweden. He presented both the results of a trial that applied AI to six years of patient data collected by a healthcare centre and its important takeaways concerning AI’s future in healthcare.
Not Just for Enormous Amounts of Data
Although AI has major potential to improve healthcare, current applications rely on very large quantities of data and large-scale training exercises to teach algorithms to “think”. What’s more, Sweden’s Patient Data Act currently limits access to large amounts of patient data for purposes other than research projects.
“Those obstacles aside, individual healthcare providers are permitted to analyse patient data that they themselves generate on an on-going basis within the framework of their QA and quality enhancement work. That being the case, we wanted to see if a relatively limited data set of this kind could provide benefits for staff and patients,” Fredrik Eriksson explains.
Reverse Logic
Fredrik continues:
“We also wanted to ‘flip the script’, so to speak. Ordinarily, you start with a theory – like that AI can diagnose a rare disease faster and in a smarter way than traditional diagnostic methods, or that it can identify anomalies in an x-ray with greater precision than is currently possible. In this case, however, we took the opposite approach: we began without any preconceived ideas about what AI can or cannot do and where it can provide the greatest benefit. Instead, we tried applying some of the most well-known algorithms – the ‘intelligence’ – to the data we had to see if they could generate anything worthwhile. Perhaps within a whole new area,” he reveals.
Collaboration with Inovia
Strikersoft carried out this AI-powered analysis in the spring of 2018 with the help of its new partner, Inovia. Inovia are AI experts and have extensive experience working with the technology in other industries, such as finance and telecom.
“Applying AI with a different mind-set – starting on a broad front and using an open-ended approach – was exciting. Usually, it’s the other way around: we have a specific aim and work within a very narrow framework to achieve it,” says Marcus Ekendahl, Founder & Chief Services Officer at Inovia. “eHealth is enormously exciting and we’ve identified experience gained in industries that have made greater progress that we can apply directly in healthcare. This case is one example where the opposite is true, however: experience gained here in the healthcare sector can be transferred to other industries instead,” he adds.
Normal Workload
The patient data used was sourced from a standard-sized local healthcare centre in Sweden with 12,000 registered patients. The centre has both a normal workload (Adjusted Clinical Groups, 0.98) and socio-economic index (Care Need Index, 1.12). In other words, it is a fairly typical local healthcare centre for a medium-sized or large Swedish city. The data set covered the past six years and consisted of approximately 600,000 data points drawn from medical records. All data were anonymized so that individual patients could not be identified.
As part of the analysis, it was also noted that only 20 per cent of the centre’s interactions with patients involve diagnosis. In fact, many interactions between patients and the healthcare centre concern purely administrative matters – patients call to reschedule appointments, check test results and suchlike.
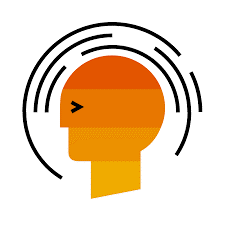
Can AI Extract Benefit from Patient Data?
Fredrik explains the challenge this way:
“The first question we asked ourselves was whether it was feasible to apply AI to the patient data at all. After all, they weren’t collected or recorded with machine processing in mind,” he says. “Secondly, could AI provide genuine added value to healthcare staff, helping them to better care for their patients?” he asks.
Another complicating factor was that the healthcare centre had changed health records system during the six-year period. All the same, there proved to be a certain commonality to the structure applied to data concerning blood tests, pharmaceuticals, diagnoses and sick leave certificates, which made it useful. This similarity was due to the fact that the centre had used Medrave throughout the period.
Applicable throughout Sweden
Fredrik continues:
“Around half of all Swedish local healthcare centres use Medrave as a tool for entering data. Consequently, if the analysis proved that AI really can provide useful information, the technique would be automatically applicable in many locations throughout Sweden,” he explains.
Automated Diagnostic Support for Diagnosis-related Groups
The next question was whether or not it was possible to establish automated diagnostic support for diagnosis-related groups (i.e., groupings of diagnoses, such as infectious diseases) based on the various data sources.
“When we talk about diagnostic support for diagnosis-related groups, we’re talking about AI providing an indication as to which type of illness a patient has based on their description of their symptoms. In other words, giving healthcare staff an indication as to where they should focus their own analyses,” Fredrik explains.
AI-powered Chatbot – Smarter with Every Dialogue
In this case, the solution applied involved combining information provided by the patient themselves (via a chatbot, for example) with historical data already recorded about them and then feeding this into the AI algorithm.
“A chatbot is a program that prompts patients to answer questions drawn from within the system, imitating a dialogue with a real person,” Fredrik explains. “What separates an AI-powered chatbot from a traditional chatbot is that it asks follow-up questions based on what it has learnt from dialogues with previous patients, and gets smarter with every answer submitted,” he adds.
How Does AI Make Sense of Medical Records?
Since the health records used contained a lot of text, much of which was unstructured, the analysis began with text processing. The first step was to “clean” the text by removing conjunctions and small words like “and” as well as commas and words often used to begin and end sentences that lack any clinical meaning.
“In this case, we made the assumption that each type of diagnosis has its own special vocabulary. By creating word clouds, we determined that the words/phrases ‘rash’, ‘cough’, ‘throat’, ‘no acute distress’ and ‘redness’ are often used in connection with infectious diseases, for example. For musculoskeletal diseases, common words used in patient records included ‘T8’, ‘T5’, ‘physiotherapy treatment’ and ‘palpation’,” says Fredrik.
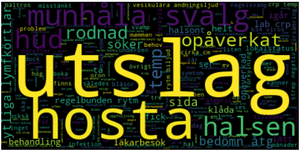
INFECTIOUS DISEASES
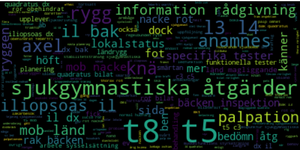
MUSCULOSKELETAL DISEASES
Leveraging Historical Data
Once the text had been cleaned up, the remaining information was combined with historical data about the patient, such as gender, age, number of visits, blood test results and vital signs. Some allowance for seasonal variation was also included. Fredrik offers some additional insight into this last variable:
“While seasonal variations shouldn’t be overemphasised, the analysis confirmed something we already knew – that certain diagnoses have a clear seasonal correlation, such as psychological illness, for example, which always peaks around Christmas/New Year and in the springtime,” he says.
The combined personal data sets were then fed into the AI algorithms, which learnt to recognise the relationships between words and categories, or diagnosis-related groups. In this case, 80 per cent of the data was used to teach the algorithm, while the remaining 20 per cent was used to test it. Finally, the likelihood that each diagnosis made was accurate was calculated; i.e. the algorithm’s accurate prediction of the diagnosis-related group as a percentage, based on the text input by the patient.
A Successful Approach
The overall result was an accuracy score of 0.62 on a scale of 0.0 to 1.0, where 1.0 represents complete accuracy. The algorithm returned a score of approximately 0.8 for the diagnoses for which it proved most accurate, while its accuracy in connection with other diagnoses was somewhat lower
“For a first attempt, an accuracy rating of 62 per cent is quite good,” Fredrik explains. “We were able to prove that using AI as a diagnostic support tool at an ordinary healthcare centre works, and that it’s a possible way forward in providing doctors and nurses with guidance when making their diagnoses,” he says in summary.
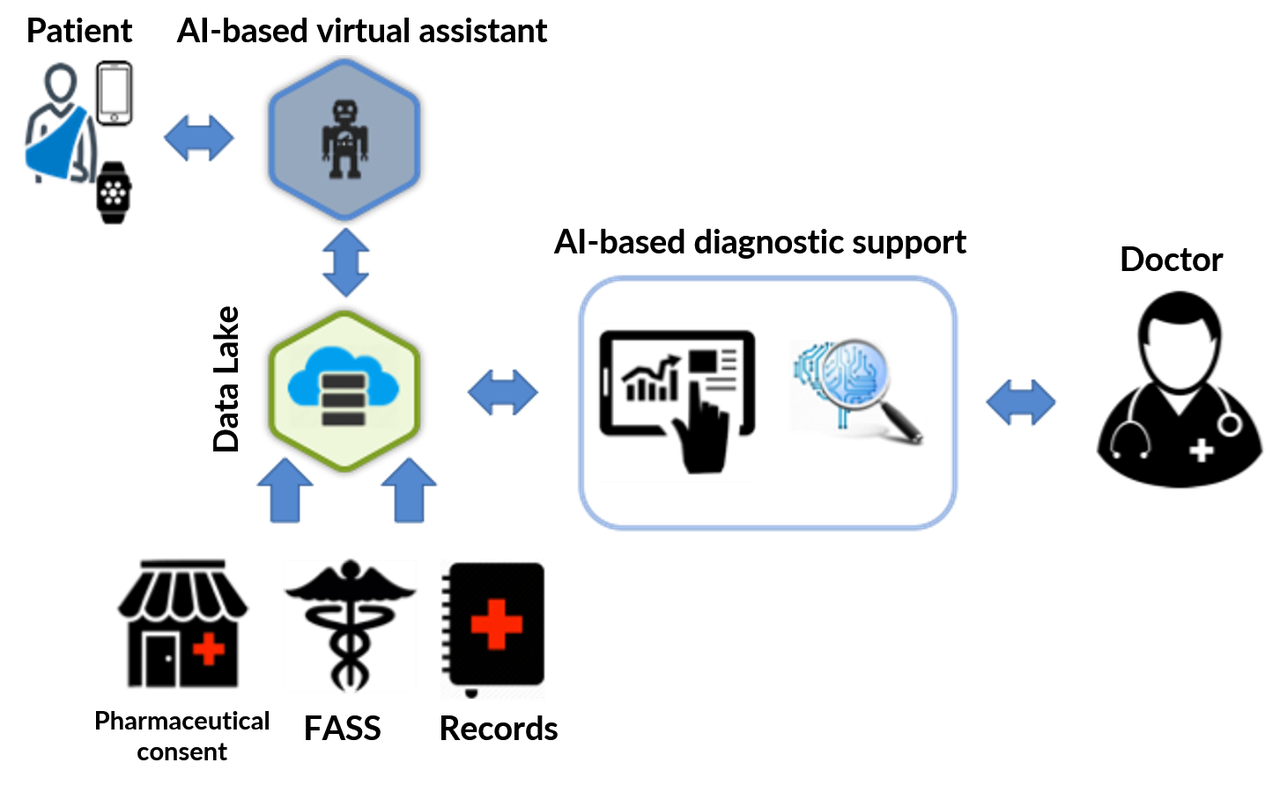
Takeaways for Improving AI’s Usefulness in Healthcare
The main takeaway from this trial is that, to ensure that the method is accurate enough to be used on a broad scale and thereby serve as a useful tool, the following are needed:
- 1. More data to help the algorithm learn and thereby increase its accuracy
- 2. A more complex model for textual analysis that includes deep learning
- 3. Preferably, the ability to use data from other sources, such as earlier health records, pharmaceutical information databases (such as Sweden’s FASS) and data that patients have collected themselves.
Feedback from Staff
The next step was to allow the healthcare centre’s staff to review the results and discuss areas where they believe AI could be an asset in their work. Their suggested areas of application were as follows:
- Allow patients to communicate with the centre before making direct contact as a means of preparing for consultations, both for triage purposes and to submit information
- Since more than 40 per cent of all calls made to the centre concern administrative matters, let AI handle these calls. A chatbot could manage enquiries about latest test results, rescheduling appointments, etc.
- Let AI handle data collected by the patient themselves, thereby promoting more patient-centred care
- Obtaining consent prior to consultations
- Retrieving patients’ medical records prior to their visit to the centre.
Relief from Administration Work
In summary, AI can help healthcare centre staff in the following ways:
- Provide relief from administrative duties by handling enquiries about appointments, test results, etc.
- Record anamneses prior to visits (phone- and video-based consultations as well as physical visits)
- Prepare for consultations by having patients update their personal data and obtaining their consent in advance. Also, directing patients to, and making appointments for testing prior to their visit
- Provide doctors with data in connection with consultations and patient interaction
- Data searches, including searches for historical data on the same patient, to help staff gain a quick overview
- Support in establishing possible and likely diagnoses based on the patient’s data, the healthcare centre’s data and external data sources.
The First-ever Primary Care Centre to Use AI?
Fredrik concludes with a positive assessment of AI’s future in primary care:
“If you look at the big picture, most of what is needed to apply AI in a healthcare centre’s operations is already in place. The processing power is relatively cheap to buy, the algorithms already exist and, if we could pool the data of 5-6 centres, we could, in fact, go live with this approach tomorrow,” he says.
Learn more about eHealth >>